The Transformative Journey of Deep Learning in Technology
Written on
Chapter 1: The Evolution of Deep Learning
Deep learning has witnessed remarkable advancements over the past six years, largely driven by enhancements in computational capabilities and algorithmic innovations. Today, it finds applications across diverse domains, including automated text generation, medical diagnostics, voice-command systems, and even weather predictions.
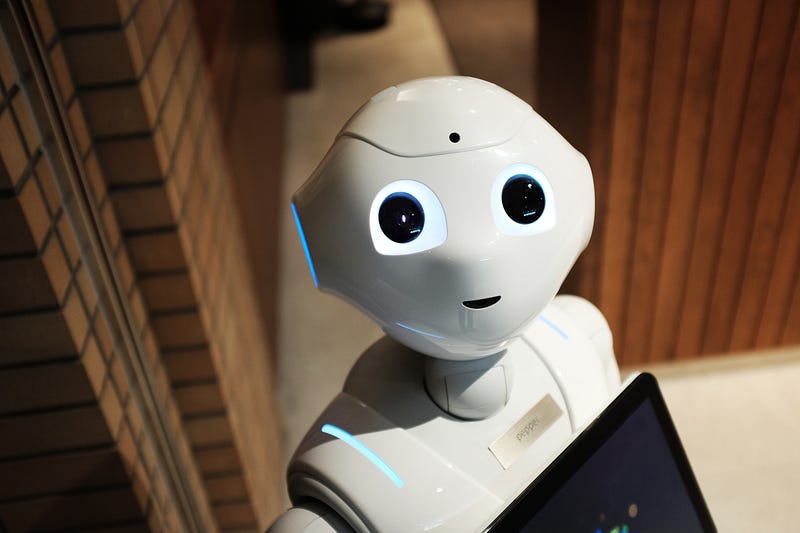
Feature Extraction: A Core Component
A pivotal element of deep learning is feature extraction, which enables algorithms to identify and process data effectively. This process utilizes algorithms that autonomously derive significant "features" from the input data. Traditionally, software developers manually selected pertinent features, but with the emergence of artificial neural networks (ANNs), this task has become automated. For instance, the initial hidden layer of an ANN may learn to detect shapes and edges, while subsequent layers might capture color information and intricate patterns.
The efficiency and speed of deep learning stand out as significant advantages. Deep neural networks leverage layers of neurons to manage data and execute a variety of tasks. However, training these networks can be challenging and time-intensive. Fortunately, advancements in high-speed computing and the availability of vast data sets have simplified this process considerably.
Section 1.1: The Vast Impact of Deep Learning
The influence of deep learning technology is extensive, permeating numerous industries. For example, commercial applications utilize image recognition and other machine learning techniques. In the medical field, it has facilitated the development of research tools, particularly for cancer detection. Recognizing its growing significance, deep learning researchers were awarded the Turing Award in March 2019 for their groundbreaking contributions.
This video titled "How Deep Learning Has Completely Changed Industries with Michael Jordan" delves into the transformative effects of deep learning across various sectors.
Section 1.2: Expanding Applications
The reach of deep learning continues to grow, particularly within the Internet of Things (IoT), which encompasses billions of interconnected devices, mobile communication technologies, and cloud services. These connected entities generate vast amounts of data that require real-time processing.
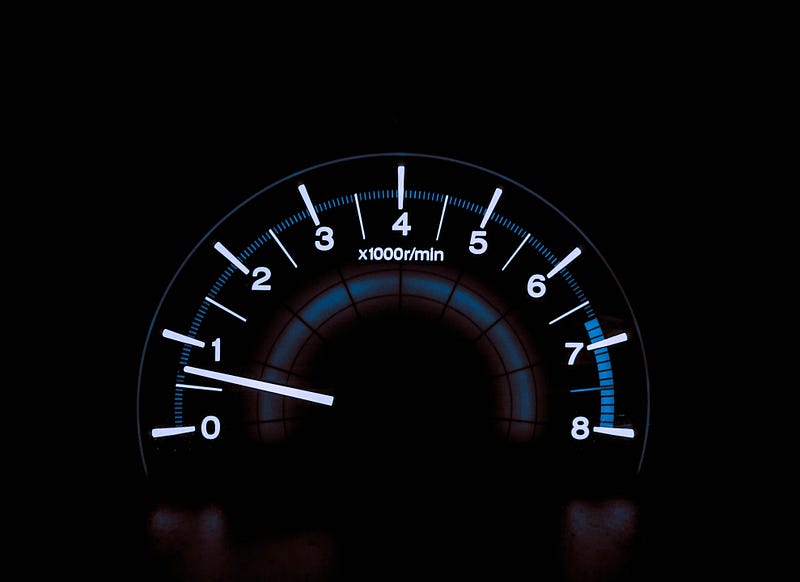
Chapter 2: The Power of Deep Learning
Deep learning, a subset of machine learning, has significantly advanced artificial intelligence. It is a robust method that employs large datasets to uncover patterns and relationships. Its applications span various fields, including automated text generation and voice search, as well as medical diagnostics.
The roots of deep learning trace back to the early 1960s when Frank Rosenblatt introduced the foundational concepts in his 1962 work, "Principles of Neurodynamics, Perceptrons, and the Theory of Brain Mechanisms." Sven Behnke later expanded on these ideas through the introduction of lateral and backward connections.
The video "AI, Machine Learning, Deep Learning and Generative AI Explained" provides a comprehensive overview of these interconnected technologies and their implications.
Applications in Various Fields
Deep learning's applications are vast, from recognizing handwritten checks to identifying fraudulent transactions. Despite its significance, many users remain unaware of the transformative potential of these technologies. This technological shift could disrupt established norms and enhance our quality of life.
In healthcare, deep learning can tackle numerous challenges, enabling doctors to concentrate on more critical responsibilities. For instance, its application in medical imaging can assist radiologists in making more accurate diagnoses and save valuable time by providing quantitative analyses of suspicious lesions.
Hardware Considerations
When implementing deep learning models, the computational power and memory required for training are crucial. A minimum of 8GB of RAM is typically sufficient for most tasks, with larger memory preferred for handling extensive datasets. An Intel Core i7 or 7th generation CPU is recommended for deep learning applications. Essential hardware components also include solid-state drives and adequate RAM.
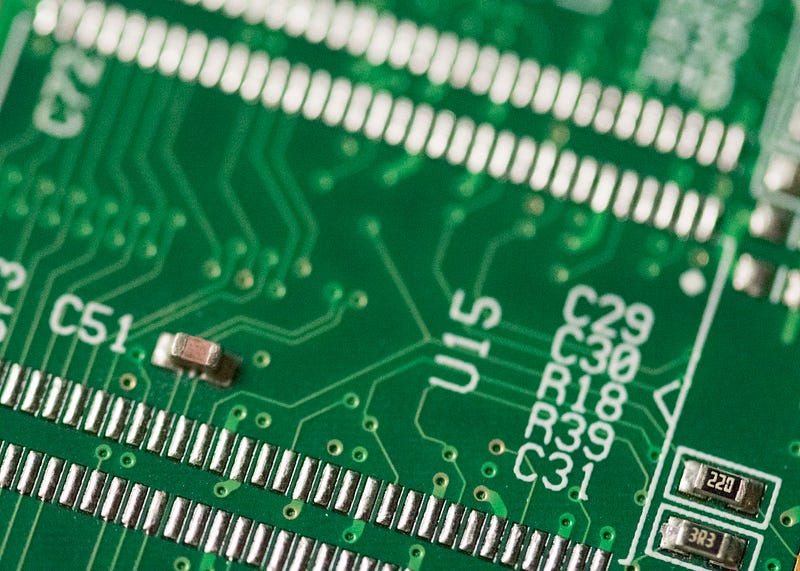
The CPU plays a vital role in managing virtual machines, distributing tasks to GPUs, and overseeing input/output operations. Current systems often utilize AMD Epyc and Xeon Scalable Platinum processors, which are optimized for accelerating inference tasks, making them ideal for production-level AI workloads.