End-to-End FP8 Pre-training for Large Language Models
Written on
Chapter 1: Introduction to FP8 Training
In the realm of training large language models (LLMs), utilizing full precision can lead to significant memory overhead. Typically, model parameters such as weights, gradients, and optimizer states are stored as float32 types, each consuming 4 bytes of memory. This results in a staggering 16 bytes per parameter, making the memory requirements for a 10 billion parameter model soar to at least 160 GB, not including the space needed for activations.
Section 1.1: Advantages of Mixed-Precision Training
By leveraging mixed-precision training, weights and gradients can be stored as bfloat16, effectively halving their memory usage to 2 bytes each. Meanwhile, optimizer states retain their float32 format. Consequently, the memory demand for the same model can be reduced to 120 GB.
Subsection 1.1.1: Exploring FP8 Data Type
To optimize further, one can quantize optimizer states to 8-bit (1 byte) using the FP8 data type, which brings down the memory requirement to an impressive 60 GB. However, it is crucial to maintain weights and gradients in bfloat16 format, as quantizing these to 8-bit can often introduce instability and divergence during training.
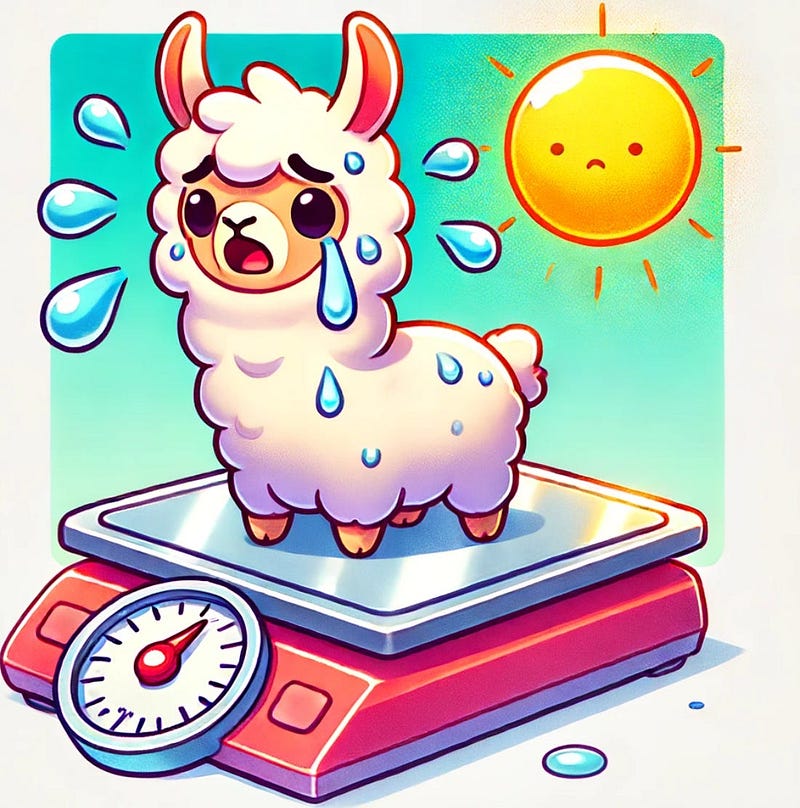
Section 1.2: Overcoming Challenges in FP8 Training
Despite the benefits, the challenge remains to manage the training pace to prevent instability and minimize the impact of outlier features in activations. Addressing this intricate issue would require extensive discussion; further insights can be found in Hugging Face's recent publications.
Chapter 2: Implementation in Nanotron
Hugging Face has made strides in creating a reliable approach to end-to-end FP8 training, which is set to be integrated into Nanotron, another framework by HF.
The first video titled "FP8 LM - Training FP8 Large Language Models" dives deeper into the strategies and methodologies behind FP8 training for LLMs.
The second video "Yuandong Tian | Efficient Inference of LLMs with Long Context Support" discusses how LLMs can efficiently handle long contexts, enhancing their usability.
For ongoing updates and insights, consider subscribing to my newsletter for articles and tutorials on the latest developments in AI:
The Weekly Kaitchup #55
Jamba 1.5 - FP8 Pre-training - Impact of Code in Pre-training Data
newsletter.kaitchup.com